ALFRED GALICHON'S

MASTERCLASSES
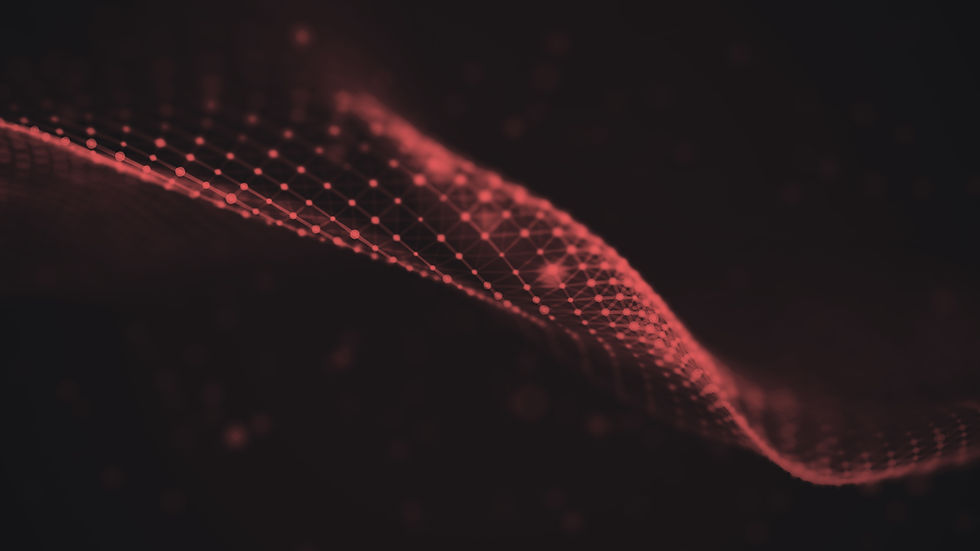
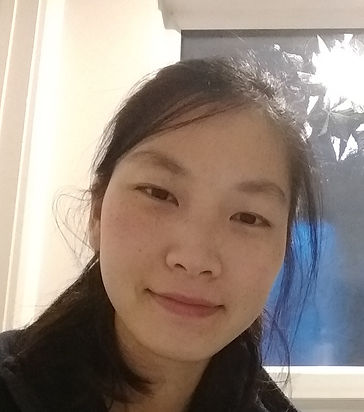
May 16, 2024
Clarice Poon
(University of Warwick)
will present:
Sparsistency for inverse optimal transport
with Francisco Andrade and Gabriel Peyré
Abstract:
Optimal Transport is a useful metric to compare probability distributions and to compute a pairing given a ground cost. Its entropic regularization variant (eOT) is crucial to have fast algorithms and reflect fuzzy/noisy matchings. This work focuses on Inverse Optimal Transport (iOT), the problem of inferring the ground cost from samples drawn from a coupling that solves an eOT problem. It is a relevant problem that can be used to infer unobserved/missing links, and to obtain meaningful information about the structure of the ground cost yielding the pairing. On one side, iOT benefits from convexity, but on the other side, being ill-posed, it requires regularization to handle the sampling noise. This work presents a study of l1 regularization to model for instance Euclidean costs with sparse interactions between features. Specifically, we derive a sufficient condition for the robust recovery of the sparsity of the ground cost that can be seen as a generalization of the Lasso’s celebrated ``Irrepresentability Condition’’. To provide additional insight into this condition, we consider the Gaussian case. We show that as the entropic penalty varies, the iOT problem interpolates between a graphical Lasso and a classical Lasso, thereby establishing a connection between iOT and graph estimation.